Tokenization: Understanding the Meaning and Uses of Tokenization in Hindi
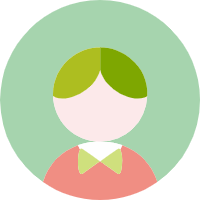
Tokenization: Understanding the Meaning and Uses in Hindi
Tokenization is a process of splitting a text into small units called tokens. These tokens are typically words, phrases, or characters, but can also include special characters like punctuation and numbers. Tokenization is essential in natural language processing (NLP) and related fields, as it helps in processing, understanding, and analyzing large volumes of text data. In this article, we will explore the meaning of tokenization, its uses in Hindi, and its applications in various NLP tasks.
Meaning of Tokenization
Tokenization is the process of dividing a text into smaller units for processing. These units are called tokens, and they can be words, phrases, characters, or even special characters like punctuation and numbers. Tokenization is important because it makes it possible to process and analyze large volumes of text data efficiently. Without tokenization, processing large texts would be time-consuming and inefficient.
Uses of Tokenization in Hindi
Hindi is a traditional language of India with a rich literature and culture. As a natural language, it presents unique challenges in terms of tokenization. However, due to the growing importance of NLP and machine learning in Hindi, there is a growing need for effective tokenization methods in this language. Here are some uses of tokenization in Hindi:
1. Sentiment Analysis: Sentiment analysis is the process of determining the sentiment or the emotional tone of a text. Tokenization is essential in this task because it helps in splitting the text into words or phrases, which can then be processed and analyzed for their emotional content.
2. Machine Translation: Machine translation involves converting text from one language to another. Tokenization is crucial in this process because it allows for efficient processing of words and phrases, which can then be translated into the target language.
3. Text Classification: Text classification is the process of assigning labels or categories to text data. Tokenization helps in splitting the text into smaller units, which can then be classified based on their content.
4. Named Entity Recognition: Named entity recognition is the process of identifying and categorizing names, places, organizations, etc. in a text. Tokenization is essential in this task because it allows for easier identification and processing of names, places, and other entities in the text.
Applications of Tokenization in NLP
Tokenization is a crucial step in various NLP tasks, and its applications are vast. Here are some examples of tokenization in NLP:
1. Sentiment Analysis: As mentioned earlier, sentiment analysis involves determining the emotional tone of a text. Tokenization helps in splitting the text into words or phrases, which can then be analyzed for their emotional content.
2. Machine Translation: In machine translation, tokenization is essential for splitting the source text into words or phrases, which can then be translated into the target language.
3. Text Classification: Tokenization helps in splitting the text into smaller units, which can then be classified based on their content. This is particularly useful in tasks like document classification or sentiment analysis.
4. Named Entity Recognition: In named entity recognition, tokenization is essential for splitting the text into words or phrases, which can then be identified and categorized as names, places, organizations, etc.
5. Chunking and Deparse: Chunking and deparse are tasks that involve grouping words or phrases together based on their context. Tokenization is crucial in these tasks because it allows for easier grouping and processing of words and phrases.
Tokenization is a crucial step in various natural language processing tasks, and its meaning and uses in Hindi are essential for understanding its importance in NLP. By understanding the importance of tokenization and its applications in various NLP tasks, one can better appreciate its role in processing and analyzing large volumes of text data. As NLP continues to grow and evolve, the need for effective tokenization methods in different languages will only become more significant.